Automating Systematic Reviews of Academic Research
Literature reviews are a critical component of evidence-based medicine, serving as a structured approach to addressing clinical questions by systematically analyzing the breadth of published academic literature. However, traditional methods require significant time, effort, and specialized expertise. This presentation introduces an advanced tool designed to automate key aspects of the literature review process. The tool offers:
- Keyword-based search across public biomedical databases.
- Advanced prompt engineering to refine criteria for paper inclusion and exclusion.
- Fact extraction tailored to extract and highlight essential data points from the target studies.
- Traceability and explainability features to ensure transparency and accountability in the results.
- A guided user interface that supports iterative refinement and validation, enabling users to fine-tune their reviews efficiently.
- This session explores the extent to which systematic reviews can be semi-automated using cutting-edge, healthcare-specific Generative AI models, and discusses the implications for the future of evidence-based medicine.
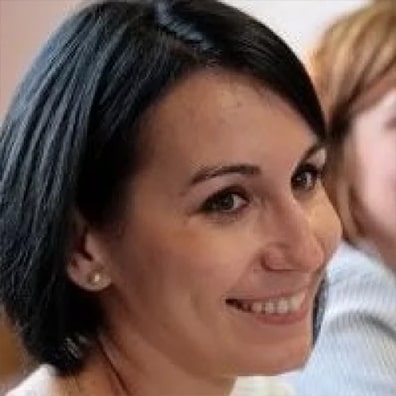
Dia Trambias
Head of Product at John Snow Labs
Dia Trambitas is a computer scientist with a specialized focus on Natural Language Processing (NLP). Serving as the Head of Product at John Snow Labs, Dia oversees the evolution of the NLP Lab, the best-in-class tool for text and image annotation in the healthcare domain. Dia holds a Ph.D. in Computer Science focused on Semantic Web and ontology-based reasoning. She has a vivid interest in text processing and data extraction from unstructured documents, a subject she has been working on for the last decade.
Professionally, Dia has been involved in various information extraction and data science projects across sectors such as Finance, Investment Banking, Life Science, and Healthcare. Her comprehensive experience and knowledge in the field position her as a competent figure in the realms of NLP and Data Science.