Detecting and Mitigating Covariate Shift for Large Language Models
Modern large language models (LLMs) have made huge progress in modeling language. However, due to the ever-changing nature of how language is expressed, language models are bound to become a snapshot of the way language was written not how it is written in the present or will be in the future. The current state-of-the-art research methods do not seem to keep track of when a language model has turned stale i.e. the model no longer represents the up-to-date text it was meant to represent. In this talk, we will first investigate this phenomena and see how LLMs fail to model unseen language.
Then, we will introduce empirically proven techniques that can help detect this phenomena in the wild. Finally, we will cover methods that can be used to alleviate the problem of covariate shift. This will increase robustness of ML systems and help NLP practitioners deploy models in the wild with confidence.
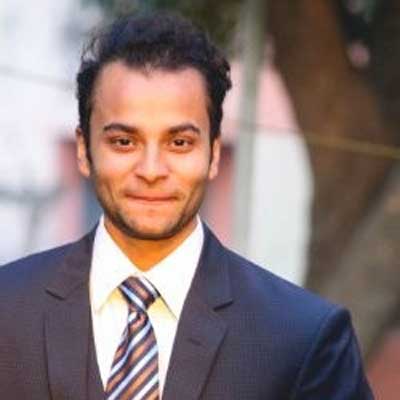
Ayush Singh
Sr. NLP ML Engineer at Cigna
Ayush Singh is a Research Engineer working at the intersection of Natural Language Processing, Machine Learning and Healthcare. He has worked on applications ranging from modeling physics of fetal brain in-situ MRI scans to style transfer in natural language generation. Recently, he has been working on using clinical knowledge graphs for query reasoning in large language models.
Ayush is driven to improve the state of education and healthcare using AI. When not working, he paints and watches cats & dogs videos on internet.