Explainable Data Drift for NLP
Detecting data drift, although far from solved for Tabular data, has become a common practice as a way to monitor ML models in production.
For Natural Language Processing on the other hand the question remains mostly open.
In this talk, we will present and compare two approaches. First, we will demonstrate how by extracting a wide range of explainable properties per document such as topics, language, sentiment, named entities, keywords and more we are able to explore potential sources of drift.
We will show how these properties can be consistently tracked over time, how they can be used to detect meaningful Data Drift as soon as it occurs and how they can be used to explain and fix the root cause.
The second approach we’ll present is to detect drift by using the embeddings of common foundation models and use them to identify areas in the embedding space in which significant drift has occurred. These areas in embedding space should then be characterized in a human-readable way to enable root cause analysis of the detected drift.
We’ll then compare the performance and explainability of these two methods, and explore the pros and cons of using each approach.
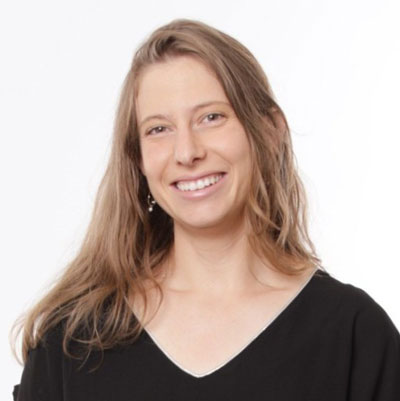
Shir Chorev
Co-founder & CTO at Deepchecks
Shir is the co-founder and CTO of Deepchecks, an MLOps startup for continuous validation of ML models and data. Previously, Shir worked at the Prime Minister’s Office and at Unit 8200, conducting and leading research in various Machine Learning and Cybersecurity related challenges. Shir has a B.Sc. in Physics from the Hebrew University, which she obtained as part of the Talpiot excellence program, and an M.Sc. in Electrical Engineering from Tel Aviv University. Shir was selected as a featured honoree in the Forbes Europe 30 under 30 class of 2021.