How Robust is Your NLP System? An Introduction to Adversarial Evaluation in NLP
In the last years there has been significant progress in a large number of downstream NLP tasks, mainly through the development of new deep neural language models. While these models increase the performance of NLP tasks, they are also more complex, more difficult to understand and, ultimately, more prone to brittleness. This is especially the case when the input these systems are applied on does not come from exactly the same distribution as the one of the data they have been trained on.
In this talk we will introduce the notion and practice of adversarial evaluation, an approach to evaluating NLP systems by exposing them to input that has been intentionally created to result in incorrect output. By applying this approach we can better understand the capabilities and limitations of an NLP system, uncover its blind spots, and avoid unnecessary risks.
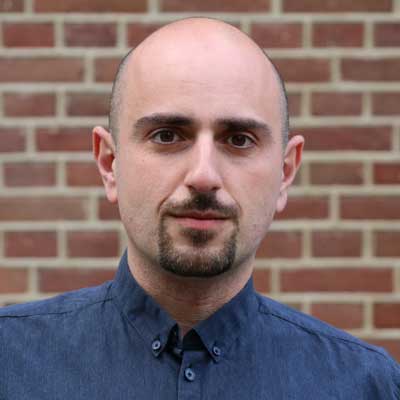
Panos Alexopoulos
Head of Ontology at Textkernel
Panos Alexopoulos has been working since 2006 at the intersection of data, semantics, and software, building intelligent systems that deliver value to business and society. Born and raised in Athens, Greece, he currently works as Head of Ontology at Textkernel, in Amsterdam, Netherlands, where he leads a team of Data Professionals in developing and delivering a large cross-lingual Knowledge Graph in the HR and Recruitment domain.
Panos holds a PhD in Knowledge Engineering and Management from National Technical University of Athens, and has published more than 60 papers at international conferences, journals and books. He is the author of the book “Semantic Modeling for Data – Avoiding Pitfalls and Breaking Dilemmas” (O’Reilly, 2020), and a regular speaker and trainer in both academic and industry venues.