Improving Precancerous Case Characterization via Transformer-based Ensemble Learning
The application of natural language processing (NLP) to cancer pathology reports has been focused on detecting cancer cases, largely ignoring precancerous cases.
Improving the characterization of precancerous adenomas assists in developing diagnostic tests for early cancer detection and prevention, especially for colorectal cancer (CRC). Here we developed transformer-based deep neural network NLP models to perform the CRC phenotyping, with the goal of extracting precancerous lesion attributes and distinguishing cancer and precancerous cases.
We achieved 0.914 macro-F1 scores for classifying patients into negative, non-advanced adenoma, advanced adenoma and CRC. We further improved the performance to 0.923 using an ensemble of classifiers for cancer status classification and lesion size named entity recognition (NER).
Our results demonstrated the potential of using NLP to leverage real-world health record data to facilitate the development of diagnostic tests for early cancer prevention.
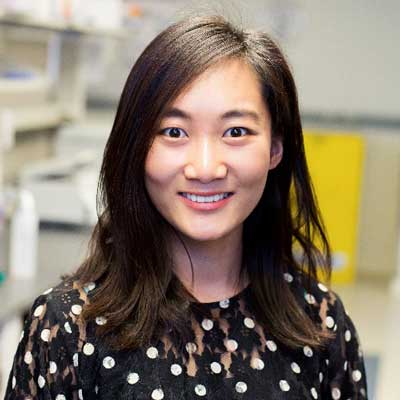
Yizhen Zhong
ML Scientist at Freenome
Yizhen’ work at Freenome centers around the development of a multi-omics machine learning model for early cancer detection through liquid biopsy.
Furthermore, she conducts research on NLP methods and infrastructure to leverage real-world data for enhancing cancer screening. She earned her PhD in Biomedical Informatics from Northwestern in 2020.
Her doctoral research concentrated on creating novel computational methods to investigate pharmacogenomics in African Americans. She served on the student editorial board for JAMIA from 2019-2020. She is passionated about application of AI methods to reduce health disparities.