Large Language Models for Parsing Clinical Text
The real-world evidence in electronic health records has the opportunity to catalyze retrospective research, but much of the data is found not in structured fields, but trapped within clinical notes. Existing techniques in clinical information extraction have often relied on having a large amount of labeled data. In this talk, I will describe how large language models can be used to parse clinical text with only a minimal amount of supervision.
I will briefly explain recent advances in large language models as well as challenges to deployment in the clinical domain. I will proceed by describing our recent work demonstrating how these models can be used for clinical information extraction, sometimes with no labels required. This covers a variety of tasks in clinical NLP, including concept disambiguation, biomedical evidence abstraction, and medication relation extraction.
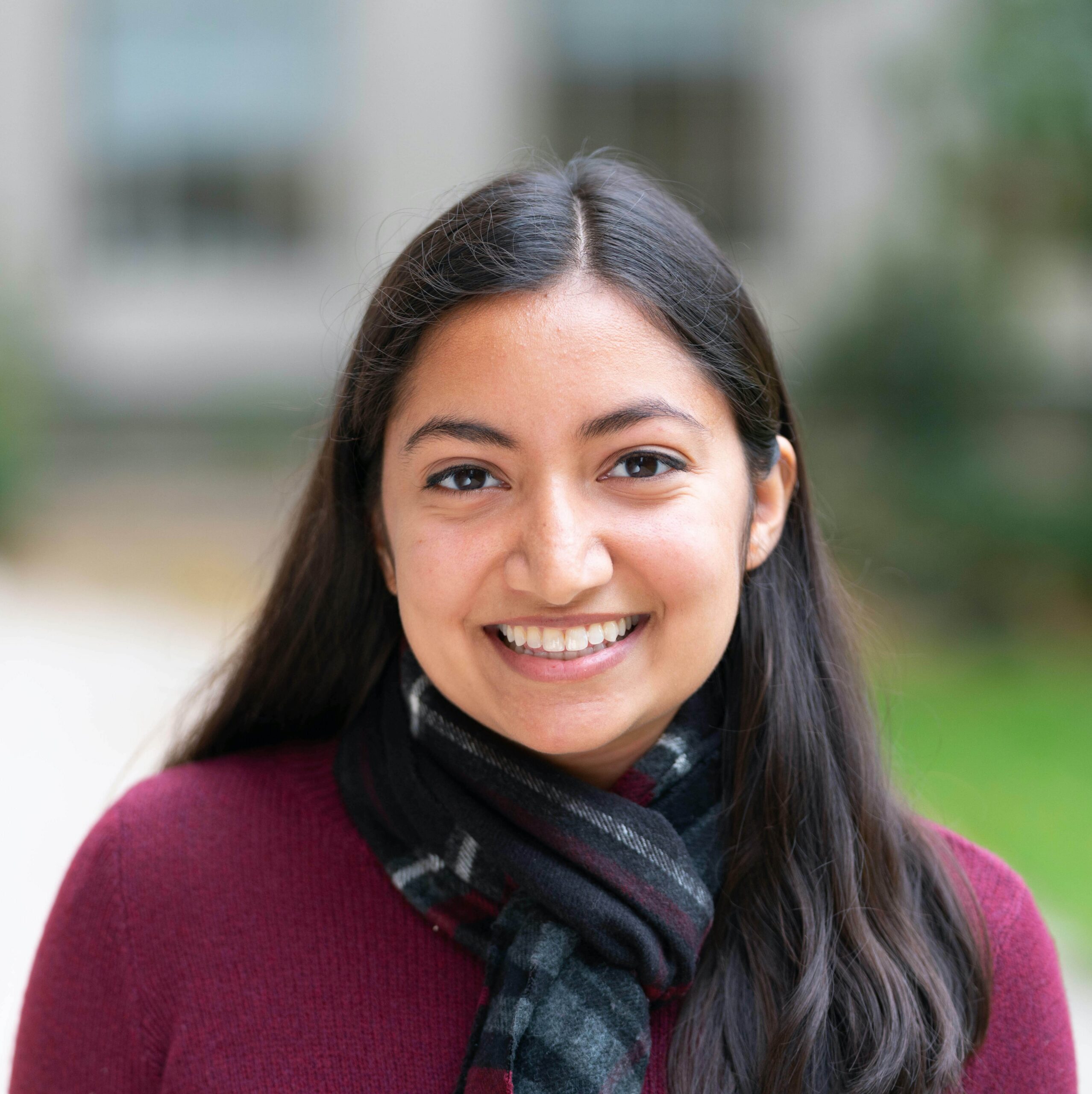
Monica Nayan Agrawal
PhD at MIT
Monica Agrawal recently completed her PhD in Computer Science at MIT CSAIL, advised by Professor David Sontag in the Clinical Machine Learning Group. In her research, she tackles diverse challenges across clinical natural language processing from few-shot learning to the design of smarter electronic health records.
Her research has been published at venues in machine learning, natural language processing, computational health, and human-computer interaction. Previously, she graduated from Stanford with a BS and MS in Computer Science.