Lessons Learned De-Identifying 700 Million Patient Notes with Spark NLP
Providence St. Joseph Health’s (PSJH) unstructured data de-identification methodology relies on pre-trained BiLSTM-CNN-Char NER models provided by John Snow Labs.
The PSJH Data science department evaluated John Snow Labs models based on accuracy and speed. The accuracy is evaluated by randomly selecting 1000 patient notes, de-identifying the notes by using the John Snow Labs de-identification model, and using human experts to validate each of the de-identified notes. There are a total of 34,701 sentences and the total number of leaked PHI events is 281.
Therefore, the PHI leaks into at least 0.81% sentences. The speed of the John Snow Labs de-identification model is evaluated by measuring the time to run 100K and 500K patient notes (expected daily load ranges from 100K-500K) using a moderate size cluster. The cluster used for this test has 15 workers, each with 112 GB memory, 1 GPU, 5DBU.
It took 43.76 minutes to de-identify 100K patient notes and 2.46 hours to de-identify 500K patient notes. In conclusion, the John Snow Labs de-identification model performs quite well as far as the speed is concerned.
The John Snow Labs de-identification model is reasonably accurate, and consistent with advertised performance accuracy.
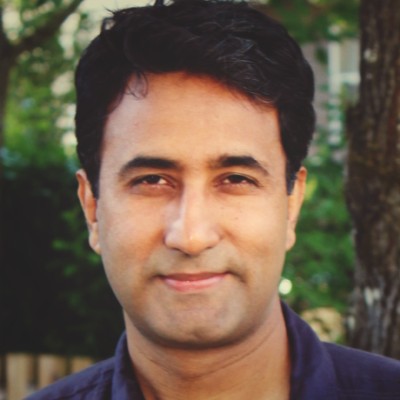
Vivek Tomer
Principal Data Scientist at Providence St. Joseph Health
Vivek Tomer is a Principal Data Scientist at Providence St. Joseph Health (PSJH) Healthcare Intelligence department where he is responsible for creating and leading strategic enterprise Data Science projects.
Prior to PSJH, Mr. Tomer was Vice President, Model Development at Umpqua Bank where he led the development of the bank’s first loan-level credit risk and customer analytics models.
Mr. Tomer has two master’s degrees from the University of Illinois at Urbana-Champaign, one in Theoretical Statistics and the other in Quantitative Finance, and has over a decade of experience in solving complex business problems using statistical models.