Long Document Abstractive Summarization with Large Language Models
Most abstractive summarization approaches of today rely on not-that-large sequence-to-sequence encoder-decoder models and are limited to contexts of 512 to 1024 lexical tokens in the source document only. In parallel, there has been significant progress in training autoregressive decoder-only Large Language Models (LLMs) with billions of parameters. These large-scale models are demonstrating impressive general language modelling capabilities, and there is a growing number of publicly available pre-trained LLMs, such as GPT-J, GPT-NeoX, OPT and BLOOM.
Can these pre-trained models be leveraged for summarizing domain-specific long documents? These models have been pre-trained on shorter documents up to 2048 lexical tokens and with general lexicon, so they require fine-tuning to efficiently tackle summarization of domain-specific long documents. However, fine-tuning LLMs on very long documents is infeasible on traditional hardware and with existing software libraries. We are leveraging industry-leading AI compute systems for this work, and we will be sharing our work-in-progress on fine-tuning autoregressive LLMs for domain-specific long document summarization.
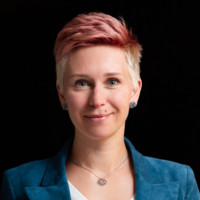
Natalia Vassilieva
Director of Product at Cerebras Systems
Natalia Vassilieva is a Director of Product at Cerebras Systems, a computer systems company dedicated to accelerating deep learning. She leads the vision and strategy for Cerebras products, market, application, and algorithm analysis for machine learning use cases. Her focus is machine learning and artificial intelligence, analytics, and application-driven software-hardware optimization and co-design. Before joining Cerebras Natalia was with Hewlett Packard Labs, where she led the Software and AI group and served as the head of HP Labs Russia in 2011-2015. Natalia also served as a part-time Associate Professor at St. Petersburg State University and a part-time lecturer at Computer Science Center, St. Petersburg, Russia. Natalia holds a PhD in computer science from St. Petersburg State University