Template Based Information Extraction with Dendograms to Classify News Articles
When dealing with several news articles, it can be cumbersome to have to classify what topic such articles are for a given website. Alternatively, one may want to discover underlying templates within diverse news categories, or even within a specific category instead. My talk deals with discovering templates from news articles in order to help classify given articles or find hidden themes.
My talk will delve with ideas I derived from Daniel Jurafsky’s paper: “Template Based Information Extraction without the Templates” along with some additional ideas I used to implement the given paper.
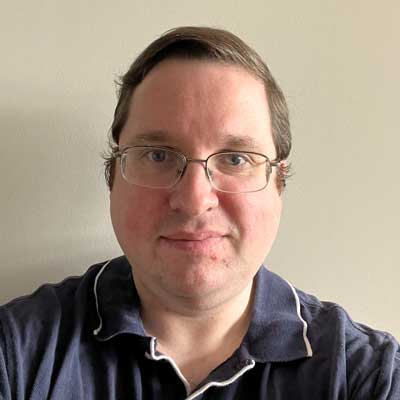
Daniel Svoboda
NLP Research Scientist Johnson & Johnson
Daniel earned his bachelors in computer engineering along with auditing a masters in mathematics from Stevens Institute of Technology in 2005. He completed his masters in Computer Engineering with a focus on Intelligent Systems (also from Stevens) in 2012. Daniel has had long experience working in NLP projects with diverse companies such as Standard and Poors, Bank of America, Verizon and AT&T. Currently, he is an NLP Data Scientist with Johnson and Johnson where he is working to automate their product selection API using natural text descriptions.
Daniel has also done some independent academic research and has a published paper along with co-authors Kevin Chen and Ken Nelson: “Use of Student’s t-distribution for the Latent Layer in a Coupled Variational Autoencoder.” Daniel is also a co-host on the podcast “Adventures in Machine Learning” where he and other co-hosts interview up and coming data scientists and engineers about the latest developments in such fields.