The State of the Art Transformer Language Models for Knowledge Graph Construction from Text
October 3rd at 2:00 PM ET – 2:30 PM ET
Knowledge graphs (KG) play a crucial role in many modern applications. Industrial knowledge is scattered in large volumes of both structured and unstructured data sources and bringing them to a unified knowledge graph can bring a lot of value.
However, automatically constructing a KG from natural language text is challenging due the ambiguity and impreciseness of the natural languages.
Recently, many approaches have been proposed to transform natural language text to triples to construct KGs. Out of those, approaches based on transformer language models are predominantly leading in many subtasks related to knowledge graph construction such as entity and relation extraction.
In this presentation, we will focus on the state of the art of transformer language model based methods, techniques and tools for constructing knowledge graphs from text, their capabilities, limitations and current challenges. It aims to summarize the research progress over the KG construction from text with a specific focus on the information acquisition branch entailing entity and relation extraction covering the state of the art transformer methods and tools. This will be useful for any practitioner who is interested in building knowledge graphs for their organizations.
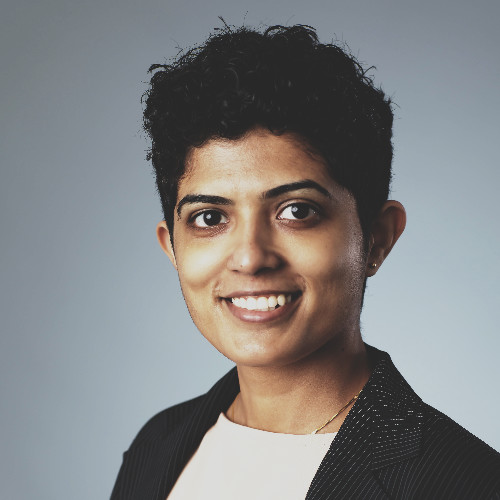
Jennifer D’Souza
Postdoctoral researcher at TIB – Leibniz Information Center for Science and Technology
Jennifer D’Souza works as a postdoctoral researcher on the Open Research Knowledge Graph (ORKG) project. There she leads the NLP services development and heads the junior AI research group SCINEXT investigating neural-symbolic AI methods for scholarly knowledge extraction and semantification.