Towards Concept-Aware Large Language Models
Concepts play a pivotal role in various human cognitive functions, including learning, reasoning and communication. However, there is very little work on endowing machines with the ability to form and reason with concepts.
In particular, state-of-the-art large language models (LLMs) work at the level of tokens, not concepts. In this work, we analyze how well contemporary LLMs capture human concepts and their structure. We then discuss ways to develop concept-aware LLMs, taking place at different stages of the pipeline.
We sketch a method for pretraining LLMs using concepts, and also explore the simpler approach that uses the output of existing LLMs. Despite its simplicity, our proof-of-concept is shown to better match human intuition, as well as improve the robustness of predictions. These preliminary results underscore the promise of concept-aware LLMs.
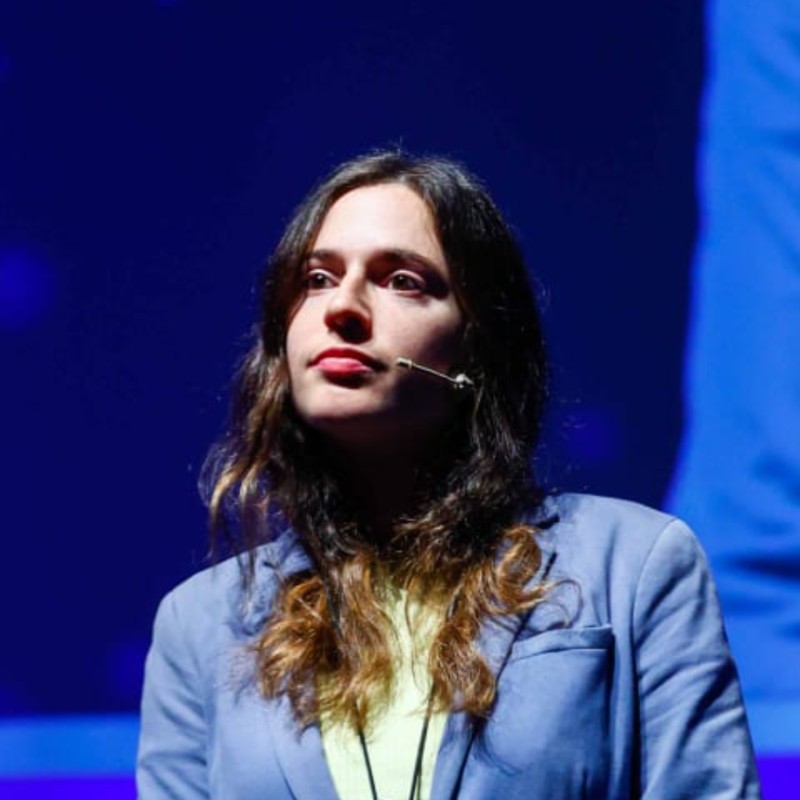
Chen Shani
Postdoc at Stanford
Dr. Chen Shani recently finished her Ph.D. in making AI more human-like (working with Prof. Dafna Shahaf from the Hebrew University of Jerusalem). She will start her postdoctoral appointment at Stanford on January 2024, working with Prof. Dan Jurafsky and Prof. Jennifer Eberhardt. Her prior education is in the fields of psychology and computational brain science. She is interested in making artificial intelligence more human-like and using it to improve human lives. She worked on enhancing Amazon Alexa’s sense of humor for over three years and then worked as an NLP consultant for startup companies.
When
Sessions: April 2nd – 3rd 2024
Trainings: April 15th – 19th 2024