Using LLMs to Build Task-Specific AI Models with the No-Code Generative AI Lab
Thousands of teams have adopted the NLP Lab in recent years to enable domain experts like doctors & lawyers to train, tune, test, and share models without coding. This talk introduces the next major iteration of this tool – the Generative AI Lab – designed for the most common use cases which organizations have asked for given the emergence of large language models: Use large language models to bootstrap task-specific models.
Enable domain experts to start with a set of prompts, for example for a text classification, entity recognition, or entity resolution problem, but then provide feedback to improve accuracy beyond what prompt engineering can provide, and have the system train a small, fine-tuned model for that specific task. This approach has the double benefit of producing higher-accuracy models than what LLMs can deliver on their own – as well as small models on which inference can be run cheaply at scale, without having to pay for an LLM service per token or for an expensive compute infrastructure. Private, on-premise, high-compliance prompt engineering. Some organizations, in particular in healthcare and finance, cannot share the documents used to train or evaluate models outside their firewall.
Additionally, the terms of use of some LLM providers do not allow using these LLMs to train downstream fine-tuned models. The Generative AI Lab comes with zero-shot prompts and LLMs that can be deployed as part of the platform, and therefore run entirely behind an organization’s firewall, with no need to call a third party API, or even have Internet access. Organize and share models, prompts, and rules within one private enterprise hub. The Lab comes with an enterprise hub that enables teams to securely share, search, filter, test, publish, import and export an organization’s private models, prompts, and rules.
This capability is designed for high-compliance enterprises to provide a central place to manage proprietary assets – including full integration with the no-code models tools (so that domain experts can publish models & prompts without a data scientist) and a full range of security controls (such as role-based access, data versioning, and full audit trails).
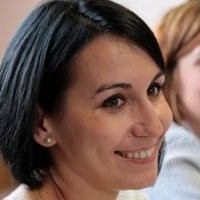
Dia Trambias
Head of Product at John Snow Labs
Dia Trambitas is a computer scientist with a specialized focus on Natural Language Processing (NLP). Serving as the Head of Product at John Snow Labs, Dia oversees the evolution of the NLP Lab, the best-in-class tool for text and image annotation in the healthcare domain. Dia holds a Ph.D. in Computer Science focused on Semantic Web and ontology-based reasoning. She has a vivid interest in text processing and data extraction from unstructured documents, a subject she has been working on for the last decade. Professionally, Dia has been involved in various information extraction and data science projects across sectors such as Finance, Investment Banking, Life Science, and Healthcare. Her comprehensive experience and knowledge in the field position her as a competent figure in the realms of NLP and Data Science.