Vision Transformers and Automatic Speech Recognition in Spark NLP
Multi-modal learning, in which a model provides answers or predictions by combining data from multiple modalities – such as images, audio, and text – is necessary in a growing number of practical use cases. This session presents new capabilities in the open-source Spark NLP library to build highly scalable pipelines that unify computer vision, speech-to-text, and text mining models.
Both training and inference will be covered, as well as the currently available pre-trained, state-of-the-art models. Finally, benchmarks that compare these capabilities to other open-source libraries will highly the order-of-magnitude speedups that Spark NLP provides for these tasks, on both CPU’s and GPU’s.
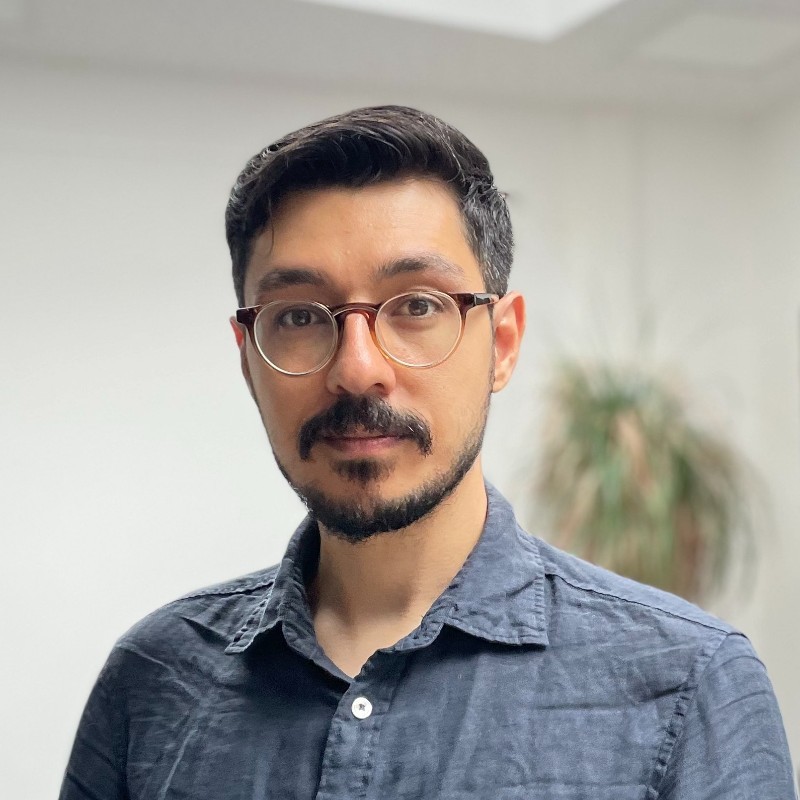
Maziyar Panahi
Principal AI/ML Engineer – Senior Team Lead at John Snow Labs
Maziyar Panahi is a Principal AI / ML engineer and a senior Team Lead with over a decade-long experience in public research. He leads a team behind Spark NLP at John Snow Labs, one of the most widely used NLP libraries in the enterprise.
He develops scalable NLP components using the latest techniques in deep learning and machine learning that includes classic ML, Language Models, Speech Recognition, and Computer Vision. He is an expert in designing, deploying, and maintaining ML and DL models in the JVM ecosystem and distributed computing engine (Apache Spark) at the production level.
He has extensive experience in computer networks and DevOps. He has been designing and implementing scalable solutions in Cloud platforms such as AWS, Azure, and OpenStack for the last 15 years. In the past, he also worked as a network engineer in high-level places after he completed his Microsoft and Cisco training (MCSE, MCSA, and CCNA).
He is a lecturer at The National School of Geographical Sciences teaching Big Data Platforms and Data Analytics. He is currently employed by The French National Centre for Scientific Research (CNRS) as IT Project Manager and working at the Institute of Complex Systems of Paris (ISCPIF).