Accelerating NLP in Production with Transfer Learning
Transfer Learning and Knowledge Distillation have accelerated the adoption of deep learning models for production workloads, particularly large language models (LLMs) that can be fine-tuned on upstream tasks that are business-specific with very little data.
Additionally, the NLP field has seen an explosion of toolsets that enable rapid prototyping and deployment of complex architectures with minimal code and general development requirements.
These high-level wrappers that abstract away the complexities are democratizing NLP and allow organizations to deliver more value, rapidly! In this talk, we explore some of the business requirements that a team of only two ML engineers has been able to deliver at SBG and some lessons learned.
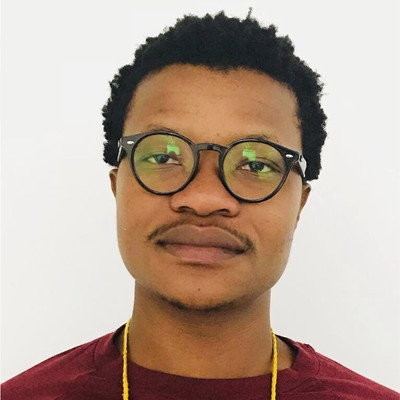
Mabu Manaileng
Lead Data Scientist at Standard Bank Group
Mabu Manaileng is a Lead Data Scientist at Standard Bank Group focusing on AI for Non Financial Risk Management. He is also a co-founder and research scientist at Datawizzards – a research lab focusing on Applied Data Science for real-world problems.
He holds a Master’s degree in Computer Science, focusing on Artificial Intelligence, Natural Language Processing. He’s an occasional open source contributor, notably for sklearn and pytorch-lightning.
His research interests include applied deep learning, probabilistic deep learning, deep reinforcement learning, on-device deep learning, graph databases, hierarchical temporal memory (HTM), and many more. Mabu was named a Top 15 Young Geek by Geekulcha in 2018 and was also listed as Top 10 Global Voice for Data Science by LinkedIn in 2019.