Combining Computer Vision and NLP for democratizing AI for Healthcare during pandemic
Machine Learning has been leveraged for a variety of medical tasks. However, much of the work has focused on feature extraction engineering which involves computing features specified by experts, resulting in algorithms built to detect specific lesions or predict the presence of many types of disease severity.
Advanced AI and machine learning techniques avoid such feature engineering by learning the most predictive features directly from the large data set of tagged examples. Identifying candidate regions in medical field is of uttermost importance since it provides intuitive hints for doctors and patients of how the diagnosis is inferred.
Natural language processing, or NLP, is a type of artificial intelligence that deals with analyzing, understanding, and generating natural human languages so that computers can process written and spoken human language without using computer-driven language.
In this talk, we explore how advanced NLP helps extract more information for the advancement of healthcare.
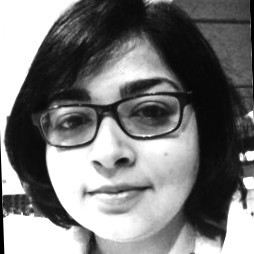
Anusua Trivedi
Lead Scientist at AI for Good Research Lab at Microsoft
As a lead scientist in the AI for Good Research Lab, Anusua works with our partners/collaborators and uses scalable and innovative machine learning algorithms for computer vision, NLP, speech, signal processing, and statistical modeling. She specifically works on advanced Deep Learning and Artificial Intelligence applications in Healthcare, where she provides leadership on complex technical problems.
Anusua is proficient in leading an interdisciplinary team for driving home a product – starting from legal reviews, related policy adherence, technical innovation, ethical AI, cyber security, and cross-collaboration across partners and academics. Anusua is a frequent speaker at machine learning conferences across the United States.
Anusua also held positions at UT Austin and the University of Utah, and currently teaches part-time at the University of Washington.