Taming the Large language models – Efficient inference of Multi-billion parameter models
LLMs have become the state of the art of generative AI and have shown promising results across different sub-fields of NLP.
Because of their extensive pre-training and at least over a billion learnable parameters, the models are sample efficient. Shows good reasoning and in context learning ability at scale.
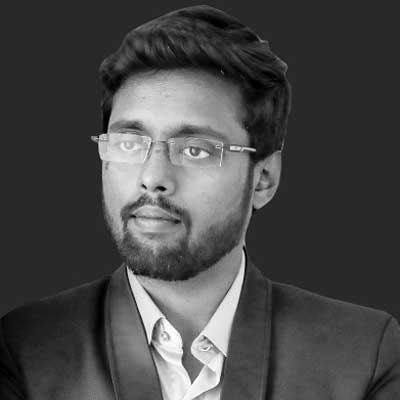
Logesh Kumar Umapathi
Lead Machine Learning Research Engineer at Saama Technologies
Logesh is a Lead Machine Learning Research Engineer at Saama Technologies, where he is leading the Machine learning efforts for a ML/NLP product aimed to accelerate clinical trials and time to market of drugs. His expertise lies in evolving deep learning-based NLP solutions from preliminary prototypes into fully functional production-ready systems. He has also published multiple peer-review research papers. His research interests include Biomedical LLMs and code generation. Apart from his professional commitments, Logesh takes an active role in the open-source community. He is a member of the Bigcode team, contributing to the efforts in building open source code generation models. Furthermore, he actively maintains ‘Mutate’, an open-source library specifically designed for data synthesis using LLMs. Outside of his professional realm, Logesh’s interests include photography and books