Utilizing NLP for Structure Learning to Understand Cancer Screening Uptake
This talk will demonstrate how NLP combined with a causal analysis approach can be used to predict and understand cancer screening uptake. Screening recommendations for breast, lung and colorectal cancer put together by the U.S. Preventive Services Task Force (USPSTF) are based on reductions in relative risk of mortality by detecting cancers at early stages where more treatment options are available. However, not all those recommended to get screened are being screened, and there are many social and economic barriers to increasing uptake. To maximize the utility of electronic health record (EHR), data in free text problem lists, as well as patient reported family histories of cancer must be extracted. Results from Stanza from (Standford NLP group) can be used with casual analysis approaches to discover underlying variable network structures, and treatment effects. However, named entity recognition (NER) alone is not quite a turnkey solution for concept extraction used in structure learning, and many challenges remain.
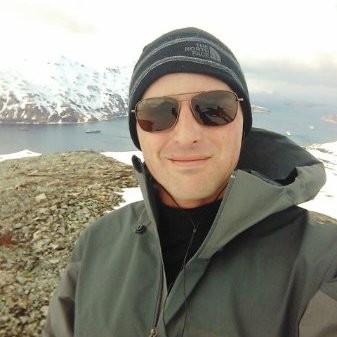
Matthew Davis
PhD Canidate in Biomedical Data Science and Informatics at MUSC
Matthew Davis is an experienced leader building AI and machine learning applications and managing data science teams with particular focus on health care applications. He currently is a Lead Data Scientist for Phreesia, and his experience includes serving as the Lead Data Scientist for the Medical University of South Carolina, as well as the Data Science Manager for the IT consulting firm Ironside. He has worked on ML applications that range from billion inference-a-year hair cut wait time models, to prediction of sepsis and heart failure readmission for hospital inpatients. He specializes in developing deep learning and natural language processing, focused on addressing complex clinical and business problems. His education background includes a BA in Aerospace Studies, and MA in Mathematics and he is PhD candidate in Biomedical Data Science and Informatics at Medical University of South Carolina. In his free time, he enjoys surfing and free diving as well as mountain biking and skiing.